Post by account_disabled on Mar 4, 2024 2:18:27 GMT -6
In today's fast-paced and changing business landscape, enterprise architecture (EA) has become a crucial tool for businesses to remain competitive and efficient. By designing and optimizing a company's structure and processes, EA can help businesses align their goals, resources, and technology to drive innovation and growth. However, the complexity and scale of modern businesses often pose significant challenges for EA practitioners. Traditional EA planning and implementation methods may need help to keep pace with the speed and diversity of business operations and IT systems. This is where artificial intelligence (AI) comes in. As a powerful technology that can automate, analyze and optimize complex tasks and data, AI can potentially revolutionize the field of EA. By leveraging AI capabilities such as machine learning , natural language processing, and predictive analytics, EA professionals can gain new insights into their organization's performance, risks, and opportunities. They can also develop more agile and adapted EA strategies to respond to changing business environments and customer needs. Innovation: How is AI used in enterprise architecture? Enterprise architecture is a method of continuous transformation of organizations, which must constantly adapt to their ecosystem: regulations, customer expectations, new technologies, etc.
This collaborative approach relies on a knowledge graph that describes enterprise components and their interactions and a software governance tool to frame and orchestrate the transformation without losing agility. In the field of EA, classic algorithms are used to perform impact analysis, comparison of scenarios or analyzes of the propagation of incidents within the company structure. But as one can imagine, a business is complex, non-linear systems involve many variables. And for such a system, it is difficult to model a priori the equations of its behavior and the rules that govern its evolution. Artificial Industry Email List intelligence, especially deep learning , plays with different levels of abstraction—hence the notion of depth—to extract meaning from the data and produce a result, freeing itself from knowing the equations that govern the system. These are some fields of application of AI and deep learning important for EA: Image recognition It allows you to photograph plans to convert them into structured models instantly. Models can be a process, data, application structures, models, computational networks, etc. Converting the plans into a structured model allows you to analyze it, for example, to know that sensitive data is being used within the framework of a specific business process.
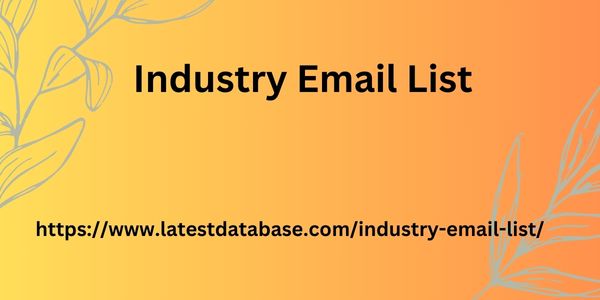
Linguistic translation Many companies need to communicate internally in different languages: an official working language complemented by local languages that allow each language community to contribute with their mother tongue. Instant or batch machine translation works great for creating a multilingual repository and facilitates internal communication and alignment. Model transformation The particularity of any model is that it is not universal. Models are representations of reality designed for a particular use. We must transform models to produce different visions for various services. A dual mechanism of formal graph transformation plus artificial intelligence can be relied on to provide opinions that address the concerns of multiple enterprise users. Natural Language Processing (NLP) (Nearly) natural language processing techniques are based on the semantic analysis of the question and its approximation to the information of the reference frame [through vector distances, for example Whe provision of results through queries and reports makes it easier for all company employees to consult the data so that they understand its operation and structure and contribute to its transformation. Data normalization On the same principle as NLP techniques, data normalization allows terms to be reconciled and possible data summaries to be presented.
This collaborative approach relies on a knowledge graph that describes enterprise components and their interactions and a software governance tool to frame and orchestrate the transformation without losing agility. In the field of EA, classic algorithms are used to perform impact analysis, comparison of scenarios or analyzes of the propagation of incidents within the company structure. But as one can imagine, a business is complex, non-linear systems involve many variables. And for such a system, it is difficult to model a priori the equations of its behavior and the rules that govern its evolution. Artificial Industry Email List intelligence, especially deep learning , plays with different levels of abstraction—hence the notion of depth—to extract meaning from the data and produce a result, freeing itself from knowing the equations that govern the system. These are some fields of application of AI and deep learning important for EA: Image recognition It allows you to photograph plans to convert them into structured models instantly. Models can be a process, data, application structures, models, computational networks, etc. Converting the plans into a structured model allows you to analyze it, for example, to know that sensitive data is being used within the framework of a specific business process.
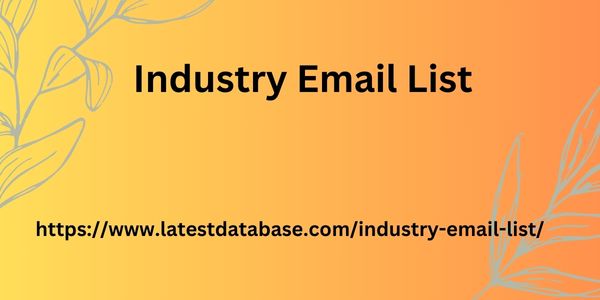
Linguistic translation Many companies need to communicate internally in different languages: an official working language complemented by local languages that allow each language community to contribute with their mother tongue. Instant or batch machine translation works great for creating a multilingual repository and facilitates internal communication and alignment. Model transformation The particularity of any model is that it is not universal. Models are representations of reality designed for a particular use. We must transform models to produce different visions for various services. A dual mechanism of formal graph transformation plus artificial intelligence can be relied on to provide opinions that address the concerns of multiple enterprise users. Natural Language Processing (NLP) (Nearly) natural language processing techniques are based on the semantic analysis of the question and its approximation to the information of the reference frame [through vector distances, for example Whe provision of results through queries and reports makes it easier for all company employees to consult the data so that they understand its operation and structure and contribute to its transformation. Data normalization On the same principle as NLP techniques, data normalization allows terms to be reconciled and possible data summaries to be presented.